How artificial intelligence is revolutionising drug design
Whether it be through music, navigation or face recognition, AI is present all around us. In this article, Shazia Absar explains how it can be used in drug discovery
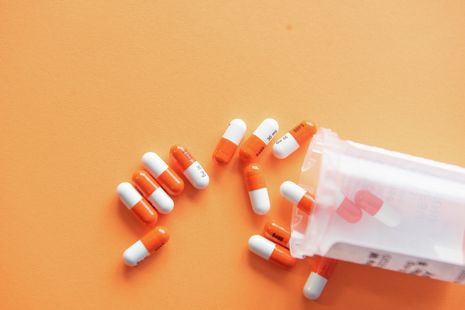
Imagine you wanted to design a drug for a new disease, ‘Disease X’, about which little is known. Imagine then that you have a machine that could use all the available data in the world about Disease X to identify a potential mechanism of disease and use this to predict which molecules within this mechanism could make suitable targets for drugs against the disease. Then, a machine would virtually design a drug targeting these optimal molecules, building it bit by bit and continuously checking with the target’s structure to ensure activity at the desired binding site. Once the drug was “built”, it could then be synthesised and, following various rounds of in vitro, in vivo, and clinical testing to validate its efficacy, the drug could be used in clinical practice.
Although a machine like this does not yet exist, advocates of artificial intelligence (AI) propose that AI has the potential to revolutionise drug design, turning this imaginary scenario — at least in part — into a reality.
Despite still being in its primitive stage, AI has become of one of the key themes when talking about the future of the pharma sector and drug design. Many new pharmaceutical companies are focusing on AI to guide their technology and design processes, and several have produced molecules that have progressed to clinical trials. The first of these companies was ExScientia, a global AI-native pharmatech company, who announced in January 2020 that its drug DSP-1181, a 5-HT1a agonist for OCD, was entering clinical trials. The exploratory research phase for DSP-1181 was less than 12 months, compared to the average of five years using traditional drug design processes. Just 11 months later, ExScientia announced that EXS21546, an A2a receptor antagonist that could be used to treat cancers, had also been developed.
“AI can reduce the time required for drug design, improve success rates and allow for cheaper discovery”
In addition to these new AI-centred companies, long-established pharma companies have also formed partnerships with AI firms. Just a couple weeks ago, GlaxoSmithKline and AI-enabled pathology company PathAI announced a multi-year drug discovery partnership, illustrating the widespread recognition of the value AI can provide in drug design.
But how does AI currently contribute? While it is not yet at the level of our imaginary machine described before, it can be used to increase the efficiency of drug design when layered into the traditional approach by reducing the time required for drug design, improving success rates and allowing cheaper discovery. Specifically, AI can be used to predict molecular dynamics and conduct “virtual screening” of drug molecules (i.e., computationally fitting molecules into protein structures to see if they fit). This reduces the costs of running expensive physical experiments and allows researchers to pre-select leads for which drugs are most likely to be effective, as well as predicting their off-target effects or toxicity.
“The worry is what may happen if these algorithms fall into the wrong hands, are reversed, and used against humanity”
Furthermore, AI can be used to identify new drug targets and binding sites on known targets, allowing the design of medicines which better interact with the target molecules. For example, the protein TYK2, a member of the JAK family of proteins, is an important molecular target for drugs treating various diseases. However, drugs that target TYK2 tend to also react with multiple members of the wider protein family, leading to many side effects and safety concerns with using these drugs. AI-enabled drug discovery recently identified a new mechanism of targeting TYK2 which appears to be at least 20-fold more selective for TYK2, offering a potential solution. Once the drug has been designed, AI can mine literature and internal data to predict optimal synthetic routes for its production, further reducing the costs and time required for the process.
The uses of AI in the pharmaceutical sector extend beyond merely the design of novel drugs; it can also be used to identify drugs that could be repurposed to treat new diseases. In January 2020, as the COVID-19 pandemic swept the globe, scientists at BenevolentAI, an AI-driven pharmaceutical company, attempted to use AI to find a drug that could help patients becoming ill and dying of the disease. Using the limited knowledge available on the virus, they set their algorithm to search over 50 million journal articles and within just four days, the algorithm identified baricitinib, a drug usually used to treat rheumatoid arthritis, as a strong contender. Two years later, its efficacy in treating COVID has been validated and the UK’s RECOVERY trial, a national study of treatments for COVID-19, found baricitinib to significantly reduce deaths. The World Health Organisation also now strongly recommends the use of baricitinib for severe COVID-19.
However, the development of AI algorithms to aid drug design is not without its risks. As we become better at treating disease and creating drugs that are both effective and non-toxic, the worry is what may happen if these algorithms fall into the wrong hands, are reversed, and used against humanity. A team working at Collaborations Pharmaceuticals investigated the possibility of this and the potential danger if this were to happen. Using the same algorithms which are usually used to predict and avoid toxicity in their drugs, they explored how the algorithm could be reversed to design toxic molecules. The algorithm was trained with an initial set of molecules and left to determine how to make the molecules increasingly deadly. Within just six hours, the model had designed 40,000 potential killer molecules, some of which were known chemical warfare agents and others of which scored higher for lethality than any currently known agents.
Therefore, whilE AI is, and will, continue revolutionising drug design, allowing the creation of more effective drugs both quicker and cheaper than traditional design processes, it is important that steps are taken to ensure these algorithms are not exploited to cause harm.
News / Varsity survey on family members attending Oxbridge
4 May 2025Features / Your starter for ten: behind the scenes of University Challenge
5 May 2025News / Proposals to alleviate ‘culture of overwork’ passed by University’s governing body
2 May 2025News / Graduating Cambridge student interrupts ceremony with pro-Palestine speech
3 May 2025Lifestyle / A beginners’ guide to C-Sunday
1 May 2025