Mental health: Probing the boundaries of AI in healthcare
Chua Huikai explores the benefits and challenges of using AI in healthcare for mental health conditions, such as depression.
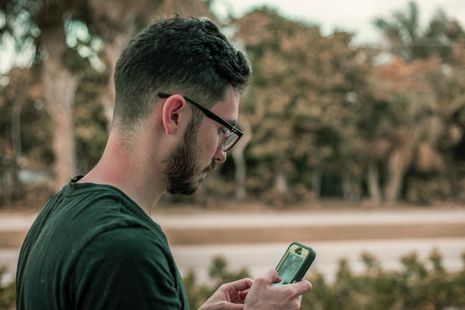
Slowly but surely, automated diagnosis using artificial intelligence (AI) has progressed beyond the realm of science fiction into reality. Researchers, companies and healthcare providers have developed and implemented AI systems to help them examine radiology scans, detect cancer, and identify bacterial infections. There is even an AI-based symptom checker available online. Much like a virtual doctor, it asks you a series of (multiple-choice) questions about your symptoms, and then suggests diagnoses which could explain them.
The common thread between these AI applications is that there is some sort of physical indication, such as anomalies in radiology scans or bacteria in blood samples, which provide hard evidence for the diagnoses that they offer. However, what about a mental health condition like depression, where most of the symptoms experienced are internal and hence unobservable? Is it still possible to apply AI to make automated diagnoses?
Some researchers think so. In the natural language processing (NLP) domain, efforts to identify linguistic cues indicative of depression have yielded promising results. For example, researchers have identified that depressed people use more self-referential thinking, especially first person pronouns, and absolutist words. What is interesting is that these can be grounded in psychological explanations. “Cognitive rigidity” has been empirically linked to depression, suggesting a relationship between the condition and black-and-white thinking (also known as splitting). Self-referential thinking can also be seen as evidence of self-focus, which has also been positively correlated with depression. Other features identified include fillers in speech, though further research has to be done on the relationship between discourse markers and depression. Further research that has been done on other aspects of human behaviour have also found differences in the way people speak. All these suggest that while we might not be able to observe the mental state of a patient directly, we can still detect its behavioural manifestations and make diagnoses based on them.
“There is the possibility of further cultural or gender bias in the datasets”
However, there are some caveats to this research. The most glaring issue, especially for NLP research, is that the findings are in English, and further research might be required to verify if they generalise to other languages. Secondly, there is also the possibility of a self-selection bias. Due to collection methods, many datasets are only able to include people who are aware of their depression and are open about it. For example, a widely-used Twitter dataset identified depressed people by looking for public tweets of diagnoses such as “I have just been diagnosed with depression”. Hence, the findings might not be reflective of all depressed people, but specifically those who have sought help for their illness, and are willing to speak about it publicly. However, it can be argued that the population researchers most wish to target, and who stand to benefit most from an automated mental health tracker are those who, afraid of societal stigma, are unwilling to share that they have been experiencing symptoms.
Finally, there is the possibility of further cultural or gender bias in the datasets. Researchers have found cross-cultural differences in the way people express depression in language, yet very few datasets differentiate between race or nationality. Algorithmic bias is an emerging problem, where AI programs are found not to be the impartial judges we hope for, but to be champions of the same human bias we exhibit. When we train algorithms with flawed data, they not only learn the bits we want them to learn – such as identifying cancer from a radiology scan – but also any bias inherent to the data. This is particularly pertinent for applications in healthcare, where prejudiced AI algorithms might have already cost millions of people the care they needed based on demographic traits. For example, as black people have been historically undertreated in the healthcare system, an algorithm trained on past data too learned to systematically undertreat an entire group of people.
Furthermore, eliminating bias in AI is not always easy. In certain applications, it might be sufficient to curate the data we feed our machines, such that they receive unbiased data and thus (hopefully) are trained to be more impartial. For example, in the previous example, we can tweak the numeric data to assign higher risk scores and hence greater urgency for treatment to black people, to match the scores assigned to white people. However, it is much more difficult to un-bias language, due to the myriad ways this can be propagated – lexically, syntactically, and so on.
“Unscrupulous companies might use it to discriminate between candidates with and without preexisting health conditions when hiring”
Another major obstacle we face when it comes to implementing automated systems for mental health diagnosis centres around privacy. One might envision a continuous monitoring system would provide the most benefits, as it can potentially detect conditions even before one is aware of it. However, would you feel comfortable allowing a browser extension to watch as you type posts on social media or message friends, or a tracker to monitor your everyday behaviour, even in the name of your health? People’s unwillingness to give away even geolocation data, via contact tracing apps, suggests otherwise. Even in Singapore, where trust in the ruling party is high, only about 20% of the population have downloaded the government-released app TraceTogether.
An ad-hoc self-diagnostic tool, similar to the symptoms checker bot from Buoy Health, might be more realistic. However, any tool – whether a tracker or checker – which allows one to diagnose mental health based on the language used by other parties still has enormous potential to be misused. For example, unscrupulous companies might use it to discriminate between candidates with and without preexisting health conditions when hiring. But how would you prove that the diagnosis you seek is for yourself? And how would you help a family member or friend unwilling or unable to enter information themselves, if you are not allowed to seek diagnoses on behalf of others? There are no easy workarounds.
The current difficult global situation has placed the spotlight on mental health. With face-to-face interactions severely restricted across much of the world, many people are cut off from their usual support networks of coworkers, friends, and extended family. Forbes has described mental health as “The Other COVID-19 Crisis”. In these circumstances, the potential benefits for a system which can automatically track mental health and remotely offer support are enormous. However, the data required to make a mental health diagnosis are significantly more sensitive and personal than that required to diagnose physical conditions. Furthermore, possible demographic bias in the underlying research has yet to be addressed. Although initial research in this area is very promising, we are still a long way off from reaping its benefits.
News / Cambridge Chancellor hopeful accuses opponent of electoral malpractice
9 July 2025News / News in Brief: Congratulations, Chancellorship, and financial challenges
6 July 2025News / Equity accuses Cambridge Shakespeare Festival of ‘wage theft’
7 July 2025Lifestyle / So, you’re dating a …
7 July 2025News / Addenbrooke’s surgeon struck off for indecent images of children
8 July 2025