How do machines translate linguistically distant languages?
Yan-Yi Lee explains how the diversity of languages creates challenges for machine translation, and how scientists might close the gap between distantly related ones
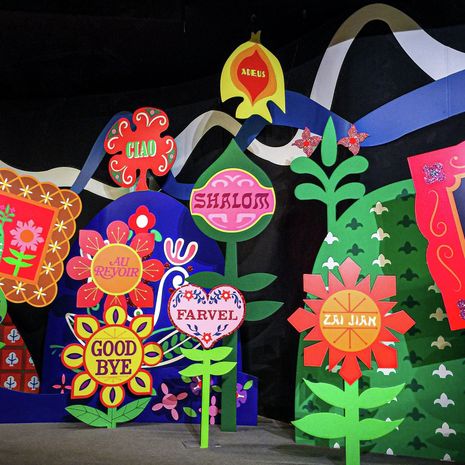
“Poisonous and evil rubbish”. “Pregnant woman over 70 lounge”. “Slip and fall carefully”. Such semantically and syntactically erroneous sentences were not taken from a practice sheet in an English language classroom, but extracted from machine translation (MT) software and published on street signs in East Asia.
Incorrect automated translations of the like trigger either raised eyebrows or giggles of ridicule, so often that meme pages have been established with the sole purpose of mocking humorous translation failures around the world (a culturally ignorant practice, but that’s an argument for another day). Beyond the superficial laughter, however, we should still concern ourselves with the issue of machine translation. In an increasingly globalised era, machine translations are destined to play a pivotal role in cross-cultural communication for generations to come. Today, while machines generally produce satisfactory translations for typologically-related language pairs (e.g. Norwegian to Swedish), problems often emerge with linguistically distant language pairs (e.g. English to Japanese). So how are scientists working to improve the latter type?
To answer that, it is first necessary to understand how machine translation functions, as well as its evolutionary path. Initially coined as computer-assisted language processing, machine translation has taken on multiple forms over the decades, each adopting a different approach to processing input and producing output. For an English sentence as simple as “The women speak with the principal”, a traditional rule-based machine translation starts with an analysis of morphosyntax (i.e. word and sentence structure). It first recognises the subject-predicate (“the women” vs. “speak with the principal”) and other key grammatical information (particularity “the” and plurality of “women”). Afterwards, it processes the semantics of the input by interpreting what each individual word means in context (is “principal” here a noun as in “school headmaster”? Or an adjective meaning “primary”?) and finally translates the interpreted input into the target language.
“In an increasingly globalised era, machine translations are destined to play a pivotal role in cross-cultural communication for generations to come. ”
While rule-based machine translation made sense during its initial conception, it dawned on scientists that this approach was mostly suited to genetically related language pairs – those that are highly comparable in terms of linguistic structure. The primary issue here concerns some of the finer dimensions of linguistic (or “typological”) distance. It doesn’t take a professional linguist to know that not all words are easily translatable due to cultural differences; nor do the same linguistic features exist across all languages. Furthermore, context-heavy languages are particularly problematic for rule-based translation software to process. The Slovenian language, for example, makes distinctions between “two” and “more than two” when it comes to plurality, thus complicating the translation of the aforementioned sentence. In Mandarin, the absence of articles, tenses, and spaces between words also renders it difficult for rule-based machine translation to understand single, out-of-context sentences. Deciphering these subtleties, as well as flexibly adapting to finer cultural-linguistic differences across distant language pairs, is not within the remit of a dictionary-like, rule-based machine translation.
The invention of statistical machine translation solved some of these issues. Working from an existing database of written work already translated by humans, statistical MT renders the resulting text more naturally. Nevertheless, its quality ultimately depends on the size of the database, meaning that less commonly translated language pairs (often distant from each other) are supported less. It is also noteworthy that statistical machine translation, reliant on existing translations, still falls victim to linguistic distance if specific patterns of the input or output phrase don’t exist in the database.
These trials brought experts to conclude that language processing is unique to the intricate workings of the human brain, and it was at this point that scientists found a promising future in neural machine translation (NMT). NMT’s novelty lies in the use of an artificial neural network to predict the probability of a word sequence. While NMT isn’t a giant leap from statistical MT, nor is it omnipotent by nature, it is nevertheless a starting point to better address the translation of linguistically distant language pairs. Former and current scholars at Microsoft have put forward the concept of unsupervised pivot translation for distant languages. Explained simply, this system takes an input text and translates it to a distant language via a series of “hops”. We can conceptualise these “hops” as transfer stops in transportation networks. Imagine someone who plans to travel from Thessaloniki, Greece, to Hokkaido, Japan. Because “Thessaloniki to Hokkaido” is an inherently long and less-travelled route, travellers would be obliged to first transfer somewhere more proximate. In a similar vein, because Danish and Galician are a distant language pair with a smaller readily-translated database to work with, the computer could produce more accurate and convenient translations through three well-supported hops: Danish to English, then English to Spanish, and finally Spanish to Galician. To program these hops in general, the learning to route (LTR) method is employed to determine the most efficient “path” for a given distant language pair.
Although machine translation may never truly parallel good old-fashioned human translation, few would deny its significance to cross-cultural communication (especially in technical fields). And while challenging issues in distant-language machine translation continue to propagate, they reflect none other than the sheer creativity, diversity, and malleability of languages around the world. And this, in my view, is genuinely something to celebrate.
Comment / Why shouldn’t we share our libraries with A-level students?
25 June 2025News / Lord Mandelson visits University
30 June 2025Features / 3am in Cambridge
25 June 2025Theatre / Twelfth Night almost achieves greatness
26 June 2025Features / What it’s like to be an underage student at Cambridge
29 June 2025