Are we really going to be replaced by robots?
Where do we currently stand in terms of technology?
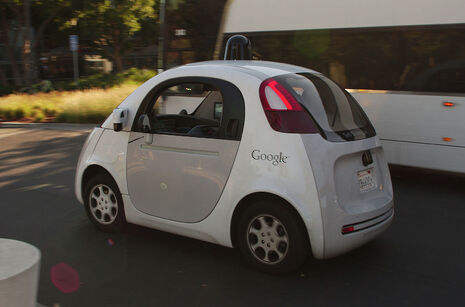
Since Alan Turing, ‘artificial intelligence’ has been synonymous with computer programmes, complicated expert systems, and simple statistical models. Today, ‘artificial intelligence’ really means deep neural networks (DNNs), and their myriad of applications.
In the last five years, neural networks have generated unprecedented improvements in image and speech recognition – often achieving better than human accuracy. Neural networks can now learn to play real-time games which don’t require planning or memory, as well as turn-based games such as ‘Go’, to a superhuman level.
It is not only the degree to which they have improved the state of the art, but also the range of tasks they have affected that makes them so exciting.
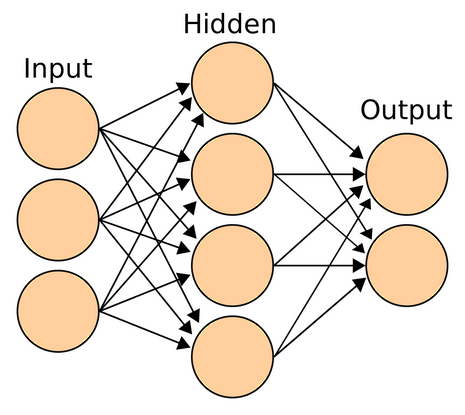
There are two applications of DNNs which are set to explode in popularity in the next five years.
Dialogue systems
Old dialogue systems like those found in adventure games use a simple decision tree, with a fixed number of allowed responses at each step. Newer dialogue systems like those provided by Amazon Echo or Google Home use the same decision tree structure, but augmented with a DNN to understand all possible variants of the usual two or three acceptable responses at each step.
Taking this further, by introducing a second DNN for speech recognition, we could conceivably automate any job which uses a telephone or messaging service to complete a form. Every pizza restaurant, hospital and customer service centre taking a large amount of traffic may opt to direct a portion of their customers to dialogue systems.
The responses of these dialogue systems, however, are still limited to pre-constructed decision trees. Artificial intelligences that can have arbitrary conversations are out of reach for now.
Self Driving Cars
Earlier self-driving cars used complicated, non-standard 3D mapping sensors. Modern iterations use DNNs to continuously convert a set of video frames and driving instructions (e.g. ‘turn left’) into a wheel direction and accelerator level. Much of the technology is present in home computers, making them much cheaper to manufacture.
Besides hardware, they require a few thousand hours of human driving data to learn from. This manifests itself as a large, but well-understood, task for a company to start manufacturing its own self-driving cars. I expect to see a lot of existing car companies enabling their vehicles with this technology.
This learning process also means that self-driving cars drive exactly like a human, although more reliably. Thus, they can be incrementally introduced onto existing roads without any additional infrastructure. What remains is simply a legislative barrier, which, once removed, will see a flooding of the self-driving car market.
A final thought concerns research. Developments in trainable robotics and general internet-roaming agents are two promising fields. AI-focused research institutions are becoming larger and more numerous, and the rate of progress is ever-increasing. But in the next five years, the impact of artificial intelligence will be measured as we take our first steps towards a new paradigm
Features / Friends, rivals, coursemates: on competition and camaraderie in Cambridge
3 June 2025News / Caius students oppose exhibition dedicated to eugenics professor’s book
5 June 2025News / John’s evicts pro-Palestine encampment with police support
4 June 2025News / Trinity and John’s seek injunctions against pro-Palestine encampment
5 June 2025News / Hospital workers stage protest as Cambridge University Hospitals face 500 job cuts
4 June 2025