Black people put at risk by healthcare data biases
Sona Popat discusses the various ways in which biases towards white people in data used in scientific research and healthcare leave Black people and other minorities in palpable danger.
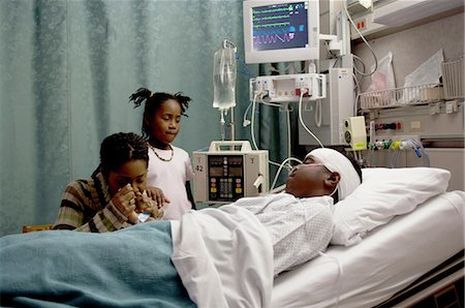
Content note: discussion of race and racism
In the UK, Black women die during childbirth at rates five times higher than white women: 40 per 100,000 compared to 8 per 100,000. In US hospitals, Black patients are 50% less likely to receive pain medication than white patients, even when comparing cases with similar symptoms or levels of pain. The racial inequalities seen in healthcare systems across the world are more than the unconscious bias of individuals. Systemic racism, where society is designed for the “average” person – an able-bodied, 30-year old white man – means people of ethnic minorities, particularly Black people, are consistently being put at risk in healthcare systems.
Medical Trials
During the drug design process, clinical trials are done on humans to ensure that the drugs are having a significant benefit on health or symptoms, as well as allowing the optimum dosage and any side-effects to be determined. Many clinical trials are done on people from majority-white backgrounds, so the drugs produced may be less effective in ethnic minorities. Researchers suggest this could be due to genetic differences that lead to differences in the way symptoms present or drugs act.
“Of the patients assigned to receive extra care, 17.7% were Black; if the algorithm were unbiased, the number would have been 46.5%.”
For example, a study reported that only 5% of the genetic traits linked to asthma in European Americans also applied to African Americans. Drugs designed based on the responses of white people mean ethnic minorities may be put at risk, and this is seen in the case of asthma: African American children have died from asthma at ten times the rate of European American children. It must be noted that the disproportionate death rates measured would also be contributed to by the fact that minority groups are more likely to be living in areas with higher amounts of pollution and a lower quality of housing, so would suffer more from respiratory diseases such as asthma. The economic divide confirms the greater need for clinical trials to include people from a range of backgrounds, to ensure drugs produced are effective by all who take them.
Genomic Data + BioBank UK
The use of genomic information in medicine is becoming more of a reality, with some genes being used as markers to detect a high probability of having a disease. For conditions such as sickle cell disease, where the genetic basis of the disease is well characterised, genomic information could be essential for detecting disease and determining the appropriate treatment. However, banks of genome sequences also contain a large data bias: most of the sequences they contain are from people of European descent. Conclusions drawn from studies linking genomic information to disease risk using these gene banks will be accurate for white people, but ethnic minorities will not receive such high-quality information. A study showed that the correlation predicted between the genome sequence and risk of schizophrenia was only accurate for white Europeans; for individuals of other ethnicities, genetic testing for disease risk will either be unavailable, or will be inaccurate.
Artificial Intelligence
Headlines such as “Rise of the racist robots” are being seen as healthcare and many other sectors increase their reliance on machine learning and artificial intelligence (AI) systems. Robots cannot be racist, but they can show the same biases as those who program them, as machine learning is only possible based on datasets that are created by humans, so contain human biases. This must be considered carefully when creating and implementing AI systems for use in healthcare, as embedding these biases will continue to exacerbate racial health inequalities.
Last week, an open letter created by Cambridge Medical Society called for the Cambridge Medical School Faculty to alter the curriculum “to better teach how diseases present in a diverse patient population”, such as cardiac disease and skin conditions. Not only is the education system failing to adequately train future doctors to correctly diagnose conditions for all patients, creating a disparity in accessibility to the correct healthcare, this same disparity is being carried over into AI designed to make diagnosis quicker and easier – and, in fact, designed to eliminate any subconscious bias of a human doctor. An AI tool created to diagnose skin melanomas had a much higher proportion of false-negative results when examining images of Black skin. It was found that this was due to the datasets the algorithm was trained from, which contained predominantly images of white skin.
“Racial health inequalities are driven by both individual and systemic biases, and both need to be combatted within and outside of healthcare.”
Similarly, US hospitals used an algorithm, created using machine learning, to refer patients of a particular threshold of illness to another programme of healthcare. The algorithm ranked each patient with a risk score based on their healthcare costs, but was found to consistently rank Black patients with lower, less severe scores, even when they had the same symptoms as white patients. Fewer Black patients were referred to the programmes of healthcare that they needed, putting Black lives at risk. Of the patients assigned to receive extra care, 17.7% were Black; if the algorithm were unbiased, the researchers calculated that this number would have been 46.5%. The use of AI could have a revolutionary impact in removing human biases against patients, but not if the AI itself is trained to have these same biases.
With the Black Lives Matter movement encouraging people to learn about and aid the dismantling of racial inequalities throughout society, it is important to remember that these have always been present, including in science and healthcare. Racial health inequalities are driven by both individual and systemic biases, and both need to be combatted within and outside of healthcare. Although it is only doctors who swear the Hippocratic oath, it is evident that scientists from many fields in STEM are having a growing impact on healthcare as the use of data science and technology increases. Therefore, it is just as much the responsibility of these scientists to ensure that data biases are acknowledged and steps are taken to remove them, so that all people are able to access the healthcare they need.
If you have been affected by any of the issues raised in this article, the following information and support is available:
- Mind: A mental health charity providing free online resources.
- Samaritans: A 24-hour mental health helpline.
- The Black, African and Asian Therapy Network: A list of free services available across the UK.
- Ways To Help: A collection of resources, including petitions and bail funds, compiled by Black Lives Matter.
- Learn To Be An Active Bystander: Learn how best to challenge antisocial and discriminatory behaviour on campus.
- CamQuaranchats Podcast: The most recent episode of the CamQuaranchats podcast where students talked about their experiences of institutional racism at Cambridge.
News / Pro Vice-Chancellor leaves Cambridge for Research Ireland
16 May 2025News / Wolfson abandons exam quiet period, accused of ‘prioritising profits’
17 May 2025Comment / Lectures are optional so give us the recordings
14 May 2025Features / A walk on the wild side with Cambridge’s hidden nature
18 May 2025News / News in Brief: quiet reminders, parks, and sharks
18 May 2025